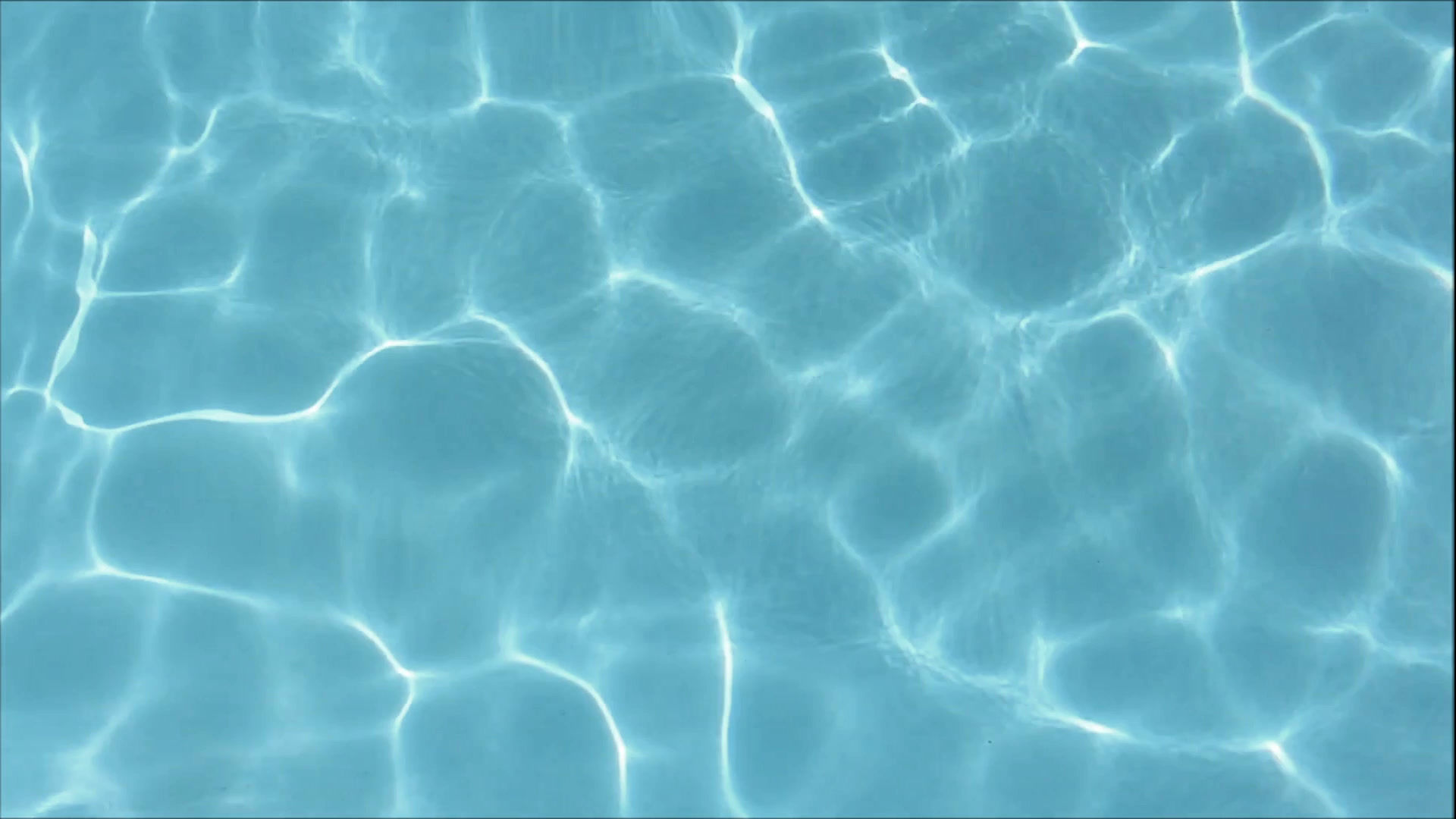
Professor (Research)
Ulsan Industrial Artificial Intelligence (UIAI) Lab, University of Ulsan, South Korea.
​
-
Condition Monitoring, Data Analysis, and Fault Diagnosis of Rotating Machines
​
In the first project, Dr. Piltan try to design and build a novel hybrid ((machine/deep) learning control) algorithm for data analysis, condition monitoring, and anomaly identification of rotating machine. Rolling element bearing represents a class of nonlinear and multiple-degrees-of-freedom rotating machines that have pronounced coupling effects and can be used in various industries. Uncertain conditions in which a rolling element bearing operates, as well as nonlinearities, represent challenges for fault diagnosis that are addressed through the fault diagnosis techniques. If defects in the rolling element bearing are not identified and diagnosed in time they can lead to the failure of the whole mechanical system. The failure of the rolling element bearing results in unexpected downtimes and great economic losses. Moreover, it can be a threat to the safety of the people working in the facility. Once the rotary machinery bearing is modeled by Dr. Piltan based on a mathematical-based modeling (e.g., five-degrees-of-freedom mathematical modeling of vibration signals) or system identification techniques (e.g., classical autoregressive technique, and machine learning based autoregressive method), and the rotary machinery bearing signals are estimated based on the extended-state observers (e.g., high-order super-twisting sliding mode observer, variable structure feedback linearization observer, and ARX-Laguerre sliding mode PI observer) or artificial intelligent based extended-state observer (e.g., fuzzy ARX-Laguerre fuzzy high-order super-twisting sliding mode observer), the decision regarding the bearing conditions can be made. In this work, machine learning-based classification techniques are employed the decision-making procedure for bearing fault diagnosis to complete the proposed techniques for diagnosis the faults by Dr. Piltan. Specifically, during the experiment Dr. Piltan drastically improve the classification challenge by up to 99.2% using learning control data analysis. Additionally, the project has yielded more than 8 journal papers and chapters since 2018.
​
​
​
​
​
​
​
​
​
​
​
​
​
​
​
​
​2. Data Analysis and Crack Size Estimation of Pipelines
​
In the second project – Data Analysis and Crack Size Estimation of Pipelines, Dr. Piltan created an intelligent technique for pipeline data analysis and crack size estimation for crack localization. Pipe networks are exposed to many difficulties such as surface load, poor quality, pitting corrosion, and water hammers which can cause cracks in the pipeline or joints. Task complexities, uncertainties, and nonlinear parameters in pipes contribute to several challenges in the detection, size estimation, and localization of leaks. Incidence leakage of a liquid from a transmission pipeline causes a sudden decline of pressure and flow rate, which can cause failure of the pipe network. Therefore, reducing the time for leak detection and identification are important to avoid product loss and other severe damage. Dr. Piltan design a robust hybrid leak detection and size estimation method based on the intelligent observation technique for pipelines. Because of the effects of gases and fluids in pipelines, accurate physical modeling of a pipeline is difficult. Consequently, the ARX-Laguerre technique is used by Dr. Piltan for pipeline modeling based on data analysis. Early detection of leaks is important to avoid product loss and other severe damage. To address this issue, the extended ARX-Laguerre PI observer is utilized by Dr. Piltan to detect and estimate a leak. In addition, a T-S fuzzy technique is applied to an extended ARX-Laguerre PI observer by Dr. Piltan to improve leak estimation in the presence of uncertainties. To leak size classification in the presence of uncertainties, the hyperbolic differential equations are governed by the T-S fuzzy extended ARX-Laguerre PI observer to find the exact solution for the kernels of a backstepping-based leak boundary. The leak estimation convergence error shows that the leak size estimation that designed by Dr. Piltan can be calculated independent of the location of the leak, which is the main contribution of this project. Regarding this research, the proposed method designed by Dr. Piltan for leak size estimation improves the delay time from 15 Second to 1 Second.
​
​
​
​
​
​
​
​
​
​
​3. Data Analysis for Active Fault Tolerant control
​
In this project, Dr. Piltan and his colleagues focused on the data analysis using artificial intelligence, modern control, and machine learning algorithms for Active Fault Tolerant control of industrial and surgical robot manipulator. Robotics industry has become increasingly important in recent years, particularly in the manufacturing and medical industries. As the robots become more complex, its importance will only grow for the world. In many ways, it is one of the most important fields of study for maintaining economic competitiveness in the future. Particularly, data analysis, active nonlinear and intelligent modern control, and machine learning algorithms deal with complex, real-world systems that modify the system output based on time-variant, dynamic input through feedback-learning in the normal and faulty conditions. An active learning-control fault tolerant control identifies the condition of data using hybrid observers to achieve a classification outcome. Moreover, an active learning control algorithm compares the actual output data with the reference data to reduce the effect of the fault. Dr. Piltan’s project dramatically improved the performance of modern control algorithm using artificial intelligence and machine learning algorithm, which is used in industrial and surgical robot manipulator. Dr. Piltan’s team drastically reduced the hand tremors problem for surgical robots and chattering phenomenon for industrial robots, which can cause increase the surgical mistakes, saturation, and overheating, through innovative application of classical algorithm. This reduced hand tremors by up to 1.5%. Moreover, his team applied artificial intelligence-based methodology, machine learning algorithm, hybrid observers and solved the controller’s dependence on the data accuracy by using those techniques for online tuning of most important parameters. Overall, the project improved the performance of detection and elimination the effect of the fault up to 98%. Additionally, the project has yielded more than 10 papers since 2018.
​
​
4. Signal/System Identification for Industrial Systems
​
In the fourth project, Dr. Piltan focused on the signal/system approximation using learning-control procedure. The main challenge in designing the procedure of learning-control data analysis is signal approximation. System approximation was divided into two principal techniques: (a) Physical-based (mathematical-based) signal modeling that is useful for prediction and (b) signal-based identification algorithm using several algorithms such as learning-control technique. In the mathematical-based signal modeling, the Lagrange technique can be used for modeling. Apart from the reliability and accuracy of mathematical-based signal modeling, this technique has drawbacks in uncertain and noisy conditions. To address this issue, the system identification techniques based on Auto-Regressive with eXogenous input (ARX)-Laguerre technique and fuzzy ARX-Laguerre procedure is used to modeling the vibration and AE signals by Dr. Piltan.
​
​
5. Full digital-based condition monitoring and data analyzing techniques
​
In this project, Dr. Piltan focused on the design, model, implementation and analysis of full digital condition monitoring system. Particularly, the research focused on Field Programmable Gate Array (FPGA), which is a high speed, low cost programmable device supporting thousands of logic gates. Applications of FPGA include digital signal processing, software-defined radio, ASIC prototyping, medical imaging, computer vision, and more. To achieve higher implementation speed with good performance, a condition monitoring and signal analysis technique was implemented on Spartan 3E FPGA using Xilinx software to monitoring and analysis the systems. The project defined a new mathematical algorithm to design a high speed digital nonlinear dynamical-base technique that improves the accuracy and increase performance. The project resulted in reduced time response, delay, and computation time.
​
​
6. Nonlinear control for industrial robot manipulator
​
Dr. Piltan led a group of 47 international researchers to design and build a nonlinear control for industrial robot manipulator in a joint project between IRAN SSP and the University of Putra Malaysia. An industrial robot is an automatically controlled, reprogrammable, multipurpose programmable in six or more axes. Robotics allow efficient production and performance with high endurance, speed, and precision. It has applications in wide range of industries such as aerospace, manufacturing, welding, painting, assembly, packaging, and inspection. Its industrial application and importance have grown steadily. By the end of 2011, there were more than 1 million industrial robots in operation, and the annual turnover for robotic systems was approximately USD 25.5 billion annually.
Dr. Piltan’s group designed and implemented a high-quality nonlinear controller for industrial Programmable Universal Machine for Assembly (PUMA) robot manipulator in the presence of uncertainties. Controller senses information from linear or nonlinear system to improve systems performance. Traditionally, industrial robot manipulators used linear methodologies. However, these methods have limited applications in complex, dynamic environments such as aerospace and surgical rooms with varying payloads or uncertainties. Nonlinear controllers are more complex, but they perform better in unknown or unstructured environments, which requires endurance, speed, and precision, because nonlinear systems can modify its outputs based on time-variant dynamic input through feedback to compare the actual output with the desired output to achieve a desired outcome.
Dr. Piltan’s project dramatically improved the industrial robot manipulators performance by improving the performance of sliding mode controller. Sliding mode controllers are commonly used in nonlinear systems as robot manipulators, but they are prone to chattering, leading to saturation, excessive heat, and over-dependence on the nonlinear dynamic formulation. Dr. Piltan introduced intelligent function that reduced the chattering and associated high frequency oscillation in a position pure sliding mode controller. Dr. Piltan’s team also designed an online fuzzy logic-based tuner to tune the important parameters to solve the dependence problem and improve precision. The project drastically improved the controller performance. The new nonlinear controller reduced the chattering up to 3%, overshoot up to 1.7%, rise time up to 1 second, and the steady state error up to 1e-3. Moreover, 64 papers were published since 2010 based on this project.
​
​
7. Intelligent tuning of fuel ratio rate in internal combustion engine
​
Dr. Piltan’s group designed an intelligent nonlinear robust control methodology fuel-ratio tuner to improve the efficiency and exhaust emissions of automobile internal combustion (IC) engines. To achieve this goal, Dr. Piltan and his team had to overcome several problems, such as modeling an IC engine with complicated nonlinear, multi inputs-multi outputs (MIMO), and time variant features; selecting the best intelligent control theory to fix the fuel-air ratio over time; and designing methods to overcome the drawbacks compared to conventional controllers. Dr. Piltan’s team carefully studied the combustion process, abnormal combustion, and cylinder pressure. They applied the Euler-Lagrange method to model an IC engine and fuzzy inference to pure nonlinear control. Then, they designed an optimal tracking method to monitor the fuel-air ratio to a desirable rate by minimizing the error between the trapped in-cylinder mass and the product of the desired fuel-air ratio and fuel mass over a given time interval. Their design successfully modelled the fuel-air ratio in a nonlinear system with up to 20% uncertainty. Additionally, the rate of fuel-air ratio error improved from 8% to 0.1%, and the rise time improved from 1.8 seconds to 0.6 second. The rate of settling time also improved from 5% to 1%. This project substantially improved the fuel-air ratio and thereby contributed the development of an efficient IC engine with less air pollution. Dr. Piltan’s research in this area has resulted in 17 journal papers and additional research in the area.
​
​
8. Filtering hand tremors in flexible surgical robots
​
Dr. Piltan led an advanced research project to reduce hand tremors in flexible surgical robots. Surgical robots in medicine have resulted in more advanced and safer surgical practices, including smaller incisions, less blood, less pain, quicker healing time, and minimum contamination. Surgical robots have also allowed access to remote areas of the body and manipulation of surgical equipment in a smaller, confined spaces within a body. Additionally, it has reduced the physical burden on surgeons, allowing a team of surgeons to operate in a more comfortable position.
Dr. Piltan and his team designed, modeled and implemented a surgical continuum robot manipulator to minimize the risks from hand tremors of surgeons operating the surgical robots. In addition to filtering the tremor, the newly designed manipulator increased the filter flexibility and reduced the system time response. A continuum robot manipulator or a “snake-arm robot” is a slender hyper-redundant manipulator. It allows higher range of motion and allows the arm to “snake” along a path or around an obstacle in a confined space. A surgical, six degrees of freedom continuum robot manipulator is a complex machine that utilizes highly nonlinear dynamic equations, MIMO (multi-input multi-out) system, time variant dynamic equations, strong coupling effects and more.
Dr. Piltan and his team designed a novel nonlinear filter to reduce the hand tremor and improve the filter flexibility. Dr. Piltan introduced feedback linearization and decoupling method to measure actual acceleration and minimize the strong coupling effects between joints and resulting tremors. His team also applied the Lagrangian formulation to design a robust stabilizing torque and introduced a new artificial intelligence methodology based on intelligent backstepping method to solve uncertainty problem and reduce the response time. This cutting-edge approach maintains the crucial balance between maximum flexibility and minimum rule-base. The minimum rule bases improved the speed rate in real time and improved the trajectory rate from 12% to 0.13% in 35% uncertainty presence. Moreover, the new design reduced the energy consumption rate from 10% to 1% and the rate of oscillation, which directly affects hand tremors, from 10% to 0.01%. Approximately 35 journal papers were published as result of this project.
​
​
9. Highly precise and dynamic controller for multi-degrees of freedom actuator
​
Dr. Piltan and his research team examined the use of new control methodology for trajectory tracking of three-dimension motors (spherical motors) for medical applications. Multi-degrees of freedom (DOF) actuators are currently used widely in aerospace, automotive, and medical industries. Dr. Piltan’s team designed and modeled a new high precision, stable and robust intelligent control unit that drastically reduced the oscillation rate from 30% to 0.01%. The new unit improved the motion precision rate by up to 40%, lowered the disturbance from 0.12 inch to 0.0001562 inch, and reduced the energy consumption rate from 8% to 0.88% and the errors rate by about 38%.
​
​
​
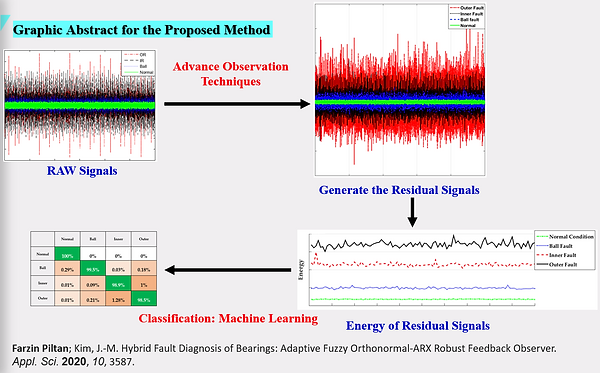
